Adam A Method For Stochastic Optimization Iclr 2015
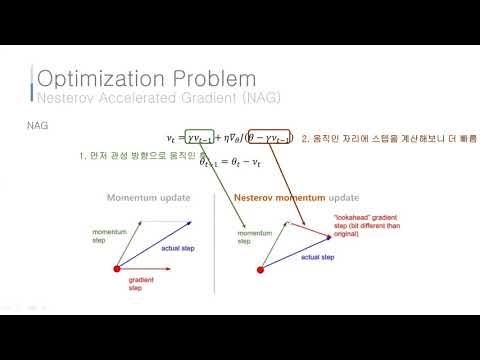
A method for stochastic optimization that the name is derived from adaptive moment estimation.
Adam a method for stochastic optimization iclr 2015. Key method the method is also appropriate for non stationary objectives and problems with very noisy and or sparse gradients. See section 2 for details and for a slightly more efficient but less clear order of computation. The method is straightforward to implement and is based an adaptive estimates. Good default settings for the tested machine learning problems are 0 001 1 0 9 8.
Please use this link for reservations. The method is straightforward to implement and is based on adaptive estimates of lower order moments of the gradients. Adam our proposed algorithm for stochastic optimization. We introduce adam an algorithm for first order gradient based optimization of stochastic objective functions based on adaptive estimates of lower order moments.
Proceedings of the 3rd international conference on learning representations iclr 2015. There is a negotiated room rate for iclr 2015. Kingma of openai and jimmy lei ba of university of toronto state in the paper which was first presented as a conference paper at iclr 2015 and titled adam. Published as a conference paper at iclr 2015 algorithm 1.
We propose adam a method for efcient stochastic optimization that only requires rst order gra dients with little memory requirement. We introduce adam an algorithm for first order gradient based optimization of stochastic objective functions based on adaptive estimates of lower order moments. An acoustic events recognition for robotic systems based on a deep learning method. We introduce adam an algorithm for first order gradient based optimization of stochastic objective functions.
The name adam is derived from adaptive moment estimation. The method is computationally efficient has little memory requirements and is well suited for problems that are large in terms of data and or parameters. Abstract we introduce adam an algorithm for first order gradient based optimization of stochastic objective functions. Note that the name adam is not an acronym in fact the authors diederik p.
A method for stochastic optimization. If you have difficulty with the booking site please call the hilton san diego s in house reservation team directly at 1 619 276 4010 ext. The method is straightforward to implement is computationally efficient has little memory requirements is invariant to diagonal rescaling of the gradients and is well suited for problems that are large in terms of data and or parameters. The method computes individual adaptive learning rates for different parameters from estimates of rst and second moments of the gradients.